- Practical AI
- Posts
- What Is Our Vector, Victor?
What Is Our Vector, Victor?
Understanding vector databases and why they are important to your AI success
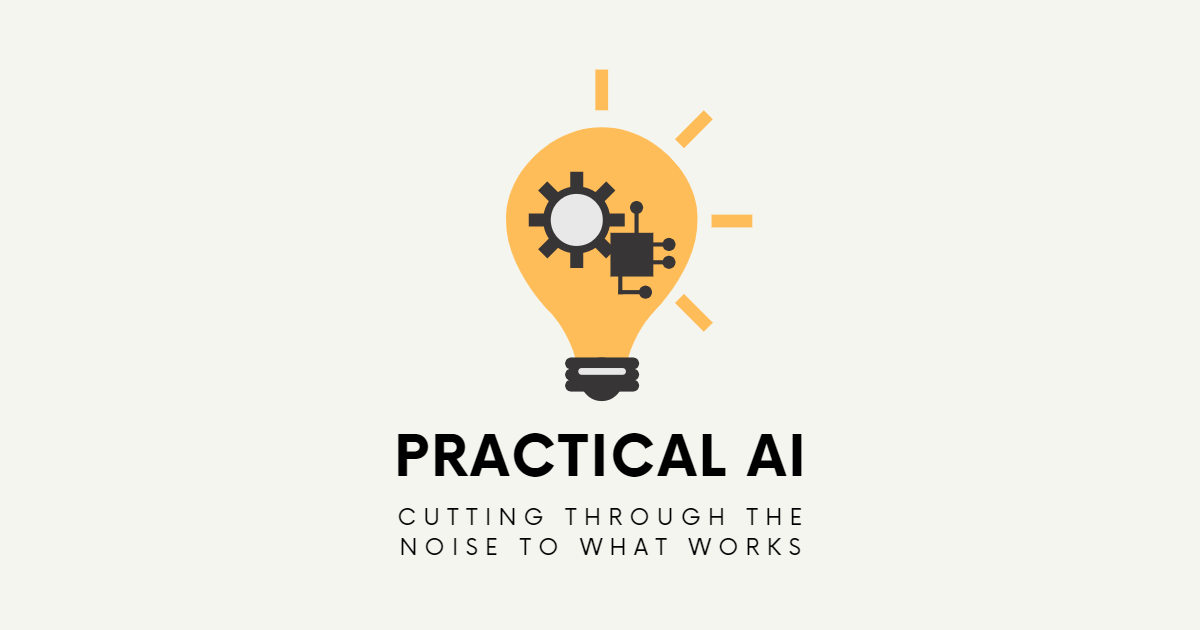
Hey there, teammate! Welcome to the next edition of the most practical newsletter on AI in the world!
Each week, I'll cut through the noise surrounding AI to serve you practical insights that not only help transform your business but also empower you, the business leader, to evolve into a data-driven, AI-enabled visionary.
The AI noise is constant and loud, and you are here for a quiet meal of AI goodness, so let’s get right to it! Bon Appetit!
Today’s Menu:
What’s Our Vector, Victor?

Famous quote from the movie “Airplane”
Action Item - Choosing the Right Vector Database
Learning Cube - Vectors, Semantic Search, Docker
Tool Spotlight - Chroma, Weaviate and Pinecone
Cool Factor - Career Essentials in Generative AI by Microsoft and LinkedIn Learning Path
July 3, 2023
In the last Practical AI newsletter, I talked about getting your own data into ChatGPT. A good introduction, to be sure, but I felt it needed (at least) one deeper dive.
So this week let’s talk about vector databases and why your choice is important. And let’s do it without hype and noise.
A vector database is where you store all of your documents and data that you want ChatGPT to search against. Each document gets a “vector” created out of it. This vector represents the theme, structure, writing style, content and many more attributes of the document.
These vectors create an apples-to-apples comparison for everything in the database! Document, image, data…they can all be “vectorized” and compared with each other.
When you put a prompt into ChatGPT, the system creates a vector of the prompt which can then be compared to all the other vectors you may have stored. The search is a “semantic search”, which means it is looking for things similar to your prompt.
There are several vector database products on the market, and surely more are coming. Each has its own specialty.
They range from smaller, local file-based databases on your machine, to large and powerful databases out in the cloud.
Since semantic searching is not 100% accurate by nature, some databases search different ways to try to get more accurate results. For example, some are great for general searching but not so great looking for software code that is similar to what you put into your prompt.
Choosing the right database for you is like choosing a car. An expensive Ferrari might be great for showing off to your social media following, but not really practical for 300 mile road trips with your family. Your minivan might not wow your neighbors but it is all you want on your road trips.
Knowing which vector database is right for you is like choosing the right vehicle: knowing what you have, where you are going, what can get you there, and what you can afford. Everything has pros and cons.
A big point here is not to get caught in the hype…either on newsfeeds or what your entrepreneur friends use. I find it pretty opinionated out there, and yet pretty obvious which vector database makes the most sense for a client's situation.
Today’s Action Item and Tools sections should help you be just as confident in your decisions as I am.

Want to feature your service or product in the world’s most practical AI newsletter? Email me at [email protected] for more information.

Action Item
Choosing the Right Vector Database
Plan. You must plan. I’ve said it every newsletter and will say it every one coming up.
AI is one of the coolest things that has ever come along and people are grasping at all sorts of shiny objects. But that can be downright dangerous for your business.
I know the noise can be loud and the gurus can make you feel stupid that you don’t know the difference between an LLM and ChatGPT.
Don’t fall prey to their posturing.
Before you start dumping things into a new Pinecone account, just because all the gurus are using it, please read this and make a plan.
I promise that you will thank me for this later.
First thing in the plan is knowing what you have. What documents and data might you need to store in a customized vector database? What will you be searching for? What data will you be correlating?
Keep it simple but have a 1-2 year plan for what data you will eventually want contained in the database, and how often you will use it. What will you be using it for? Creating SOPs? Researching changes in your customer buying patterns?
You also have to look at your data security and privacy considerations. Do you have a data security office that would not want any of your data out in the cloud? Would encryption of data be sufficient? What about customer data? You have to know if you need to scrub personal info out, encrypt sensitive data, or are even allowed to add data to a cloud database at all.
That last one sucks to have to consider and go through. It is tedious and confusing. However, based on where things are heading with lawsuits against OpenAI as I type this, it will be a major consideration for the next year or two going forward. (I expect the vector database landscape to change dramatically if lawsuits rule the day)
Armed with all of the above,(Yes, my friend. ALL of the above. Don’t skimp. It isn’t much info to be armed with), look at the Tools section of this newsletter and choose the vector database that applies most closely to you. As always, this is NOT an exhaustive list, and there are more databases coming out all the time. These are just the most popular vector databases out there. (Popular is good during fast-moving and chaotic times like this, as others will come and go and you don’t want to be stuck with something that had promise but never lasted)
Keep your answers from this section and revisit every quarter to make sure you are adapting your technology to your changing needs.

Learning Cube
Vectors
Not Vecna, the villian in Stranger Things. But vectors. These are more boring, but less nightmare-inducing.
A vector is a set of numbers tied together into one thing that represents something else. In simple terms, a vector is an arrow going from you out to some other area. It points in some direction, for some length.
There is a vector from each of us to the top of the Empire State Building. Someone close to you will have a very similar vector. Someone across the world might have the same length vector but it points in a totally different direction.
So when you are searching for people near you, you could compare the Empire State Building vectors we all have. Someone with basically the same direction and length as you will be pretty close by you! You could be different ages, genders, and like different food and music. But as far as the Empire State Building is concerned, you are very close.
The Empire State Building is one attribute. Gender, race, family medical history, height, age, clothing preferences, Netflix or Hulu subscriber, bike rider or uses a wheelchair. Imagine literally thousands of attributes. Each with a magnitude. Magnitude is strength of the attribute. Think dark green vs light green…the light green is short magnitude for “green” and the dark green is a long magnitude for “green”.
Each LLM has thousands of attributes per vector that it uses to determine magnitudes for any content you give it. Then as a whole unit, one vector is assigned to that content. The vector is the collection of the attribute magnitudes for that content. Now you can compare vectors.
You don’t have to go to dozens of data sources, query each one, determine what stays and what can go, etc etc.
You just do a semantic search on your prompt’s vector and see what is close to it.
Semantic Search
Semantic Search is how your prompt is used to search all the vectors in your vector database.
We don’t search for “Who is as close to the Empire State Building as Greg is?” We make a vector out of that question and run it through a vector database search to see what is pretty close.
A semantic search is not meant to be 100% exact. It goes against thousands of attributes and finds ones that are close to your prompt. It can be based on tone, content, previous questions and answers in the chat.
You will most likely get a different answer each time because it is looking for things LIKE the prompt. And ChatGPT does not spit back exactly what it finds, but instead takes all the vector data and creates a conversational answer for you.
So if there is a room of 10 people, and someone asks “Who is as close to the Empire State Building as I am?” then ChatGPT could say “Several people are as close as you are — person #1 for example” or “Person #7 is as close to the Empire State Building as you are.” Different wording, different people mentioned, both equally accurate from a semantic search perspective.
This is why having a vector database is so important. And also why having it handle specific types of prompts better is important. You want the best answers coming from your LLM, and that depends on what questions you are asking and what results you expect to get back.
Semantic Searching is the same idea, but how each LLM performs it, what it focuses on, can be distinctly different.
Docker
I have not discussed this much in this newsletter but it applies heavily to some of these vector databases. You simply need to understand what it is so when you read about it in the news and documentation, you can understand the ramifications.
Docker is a programming thing. It is a container in which programs can run. Kind of like your own mini server and operating system.
You can drop a vector database in a Docker container, and copy that container around. Whoever gets the container, gets not only the database but the operating system, settings, and necessary pieces to run it. The program runs inside the container. Way better than copying the database over to someone only to find it doesn’t run the same way over there.
Docker containers are self-contained and can easily be copied around.
It is obviously much bigger than that, and much more complex on the inside. But when you hear of things running in a Docker container, picture a fully contained place for a program to run — and that can be given to anyone that can host a Docker container. Pretty powerful stuff.

Tool Spotlight
Chroma, Weaviate and Pinecone
In order to quickly process, and efficiently store, your documents as embeddings, several companies have brought out vector database offerings.
Chroma, Weaviate and Pinecone — These 3 are the current big names in the vector database world. There are more than these and more will come out. (Side note that I have used Google Sheets as my vector database in small use cases just fine)
One honorable mention would be for SingleStore. It is also a popular vector database with a lot of great qualities. I don’t highlight it here because it is more than what is needed by the newsletter readers I am trying to serve.
Chroma is really the database of choice for me, and what I would likely recommend for many of you.
Good for general querying, aggregation and correlation
Local, so it is not out in the cloud where InfoSec people get uncomfortable
Also makes it easy to share with others
Can also make it hard to share with people who are not on your network
Because it runs on your machine, or a local machine, its performance can depend on machine specs, especially memory usage
Easy to make many, smaller vector databases with specific role usage such as an HR database, a CEO database, a Dev database, etc
Easy to set up and easy to use
Open source, and full-featured; well supported
Hosted cloud version is under development
Weaviate is an excellent, cloud-based or local vector database.
Good for Machine Learning, data scientists and software engineers due to ML capabilities and familiar UX to other applications used over the years
Cloud-based so easy to share and re-use
Can run locally in a Docker container instead
Easy to set up (slightly more to think about with Docker solution)
Local running is a plus, especially for the InfoSec folks
Local running performance is dependent on the machine used to host the Docker container
Supports hybrid search techniques, combining keyword-based search with vector search for state-of-the-art results
Has hooks into things like GPT-3.5.
Has more capabilities than Chroma but also has a learning curve to get into that power
Pinecone is another excellent, cloud-based vector database. This one is very popular with the gurus.
Good for high-dimensional vectors (ie. lots of attributes), performing fast and efficient searches. Optimized specifically for querying and storing embeddings like we are talking about.
Does long-term memory of search results for high-performance AI applications
Cloud-only solution
Has a simple API to hook your stuff into it
Its performance gives you better real-time analysis that other databases cannot give
Best for full-on AI, not just general querying or content generation

Cool Factor
Last newsletter we talked about Google having a learning path for AI, and this week we are talking about Microsoft and LinkedIn creating a similar, free learning path.
Interestingly, this learning path has a distinctly obvious leaning towards thoughtfulness and ethics.
It is a smaller group of courses than Google provides, but has hours of learning that can help you navigate all that is going on in Generative AI, and has a handy certificate you can earn at the end.
In any event, I highly recommend going through the learning path just so you get the big picture better, and can begin to think like an AI-enabled visionary and entrepreneur!

Feature your service/product in the world’s most practical AI newsletter
Practical AI is the world’s most practical AI newsletter with subscribers from many different industries and countries, all looking to make use of tools and services to bring AI into their businesses. You can book your ad spot by emailing me at [email protected]
In Closing…
What did you think of this week’s newsletter?
Your feedback helps me create better content for you!
Just reply back to this, or email me directly at [email protected], and let me know what you think:
5 Stars - Loved It!
3 Stars - Meh…not bad
1 Star - This sucks
If you want to sign up for this newsletter or share it with a friend, you can find us right here
Thanks for reading. Let me know if you applied anything from this newsletter!
See you next week!
Greg